Challenge
There are many variables to be considered, and a lot more data sources to integrated with in order to:
- Analyse User Behavior Analysis
- Develop Natural Language Processing (NLP) models
- Real-time Transaction Monitoring
- Anomaly Detection
- Machine Learning Model Adaptation/Evolution
- User Education and Feedback Integration
Approach
Harvest information from transactional systems against data lake:
- Extract data from transactional systems
- Apply data anonymization and cleaning
- Apply data transformations to perform aggregations, time series analysis and additional features
- Developed custom AI/ML models to detect fraud.
- Continuous evaluation of model performance to be retrained frequently to avoid decay
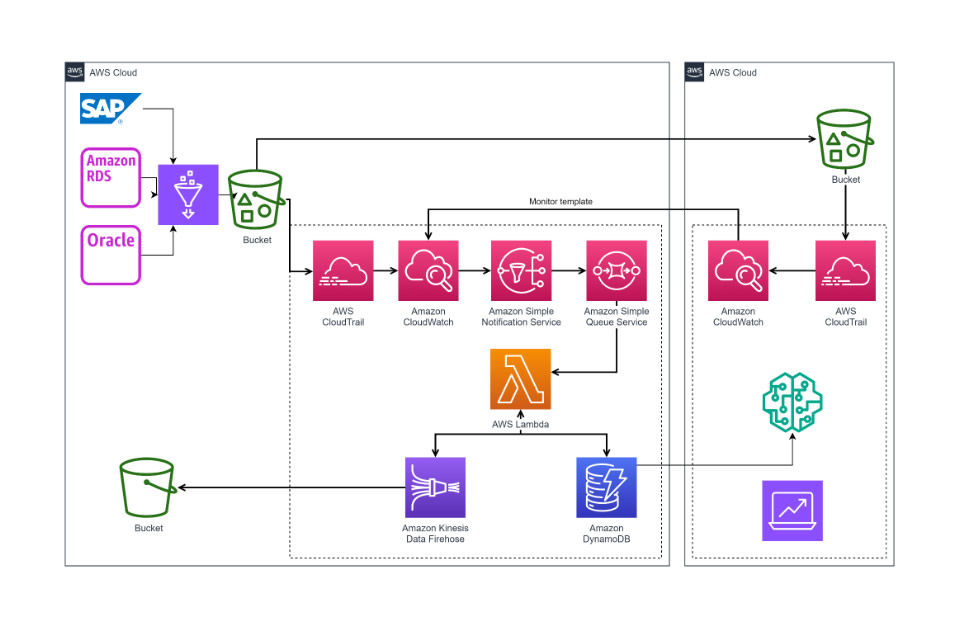
Value Delivered
- Enhanced Security: Provides robust protection against a wide range of impersonation scams, safeguarding users’ financial assets and personal information.
- Improved User Trust: Increases confidence in online banking applications, encouraging the use of digital banking services.
- Reduced Fraud Losses: Minimizes financial losses due to fraud, protecting both the users and the financial institutions.
- Adaptive Fraud Detection: Keeps pace with evolving scam tactics, ensuring long-term resilience of online banking platforms against impersonation scams.
- User-Centric Approach: Balances security measures with user convenience, ensuring that fraud prevention does not impede the banking experience.
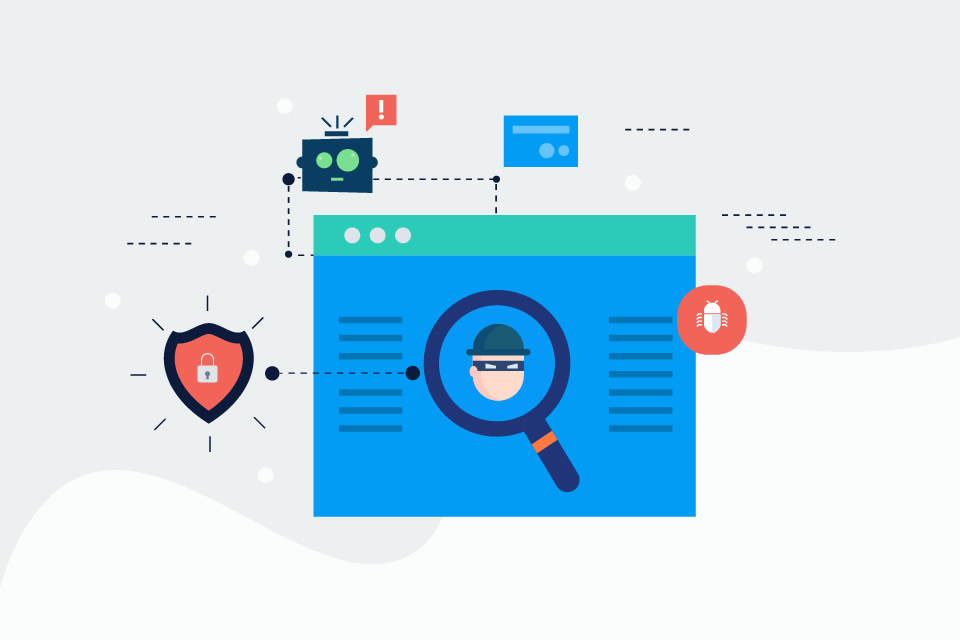
Cloud Experts
Guidance and hands-on-keyboard through your cloud journey, from migration to AI in production
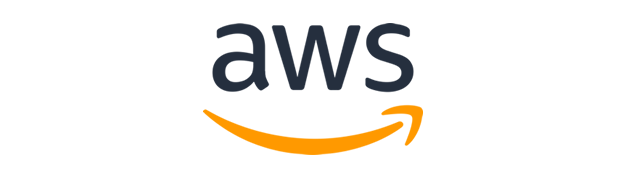
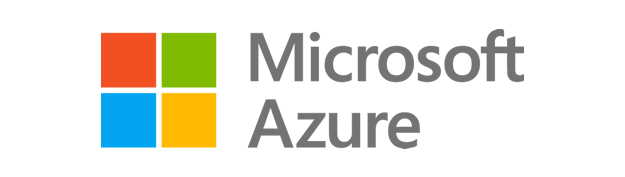
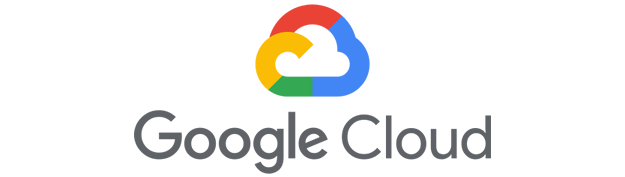
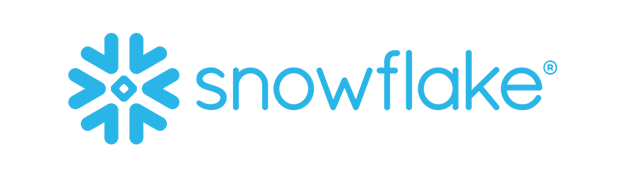
Head Office
Rua Ramalho Ortigão 213
4900-422 Viana do Castelo
Portugal
Phone: +351 258 121 902 (Call to national fixed network)
Mobile: +351 964 367 684 (Call to national mobile network)
Email: account@valuedate.pt